AI Risk Assessment and its Impact on the Insurance Industry
- Tyrone Dugmore
- Nov 6, 2024
- 9 min read
Updated: Dec 4, 2024
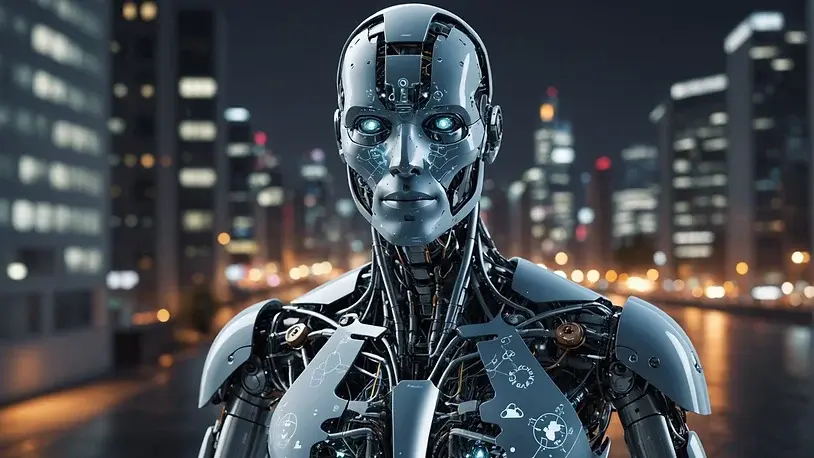
The insurance industry is undergoing a profound transformation, driven by technological innovation. At the forefront of this evolution is AI-powered risk management, which is revolutionizing how insurers assess risks through the Risk Management Framework (RMF). By harnessing advanced technologies and analyzing vast datasets, insurers can enhance operational efficiency, personalize policies to meet individual needs, and significantly improve the customer experience.
However, this shift also brings notable challenges. Concerns around data privacy, algorithmic bias, and high implementation costs present significant hurdles for adoption. To address these issues, frameworks such as the NIST AI Risk Management Framework, released in January 2023, offer valuable guidance for implementing responsible and trustworthy AI systems. These frameworks emphasize fairness, transparency, and accountability, enabling insurers to navigate the complexities of integrating intelligent technologies effectively and ethically.
In this article, we’ll delve into how advanced analytics is reshaping the insurance sector, explore its transformative benefits, and discuss critical considerations for successful implementation.
What is AI Risk Assessment?
Risk evaluation powered by artificial intelligence utilizes advanced technologies such as machine learning algorithms, predictive modelling, and big data analysis to quantify exposure and potential loss. These systems process vast volumes of structured and unstructured data, uncovering insights and precision levels unmatched by traditional methods.
Traditional vs. Modern Risk Management
In conventional insurance, assessments rely heavily on historical data, such as credit scores and past claims. While effective to a degree, this approach often overlooks real-time behaviors or environmental changes.
Modern systems take a more comprehensive approach, incorporating:
Telematics data: Insights from real-time driving behaviors in auto insurance.
IoT device readings: Data from smart home sensors for property coverage.
Behavioral analytics: Sentiment and activity patterns derived from online interactions.
This multidimensional analysis generates profiles that are dynamic, accurate, and responsive to changing conditions.
Key Benefits of Advanced Analytics in Insurance
1. Enhanced Precision
Modern tools provide exceptional accuracy by identifying patterns within real-time data that human analysts might overlook. For example, during the COVID-19 pandemic, insurers used predictive models to adjust life and health premiums dynamically based on infection rates, policyholder health data, and hospital capacities.
Potential Concern: Over-reliance on automated systems could result in errors if algorithms are poorly trained or systems fail.
Solution: Regular system updates, thorough audits, and incorporating human expertise ensure accuracy and reliability.
2. Improved Operational Efficiency
Automation reduces the burden of tedious processes like data collection, underwriting, and claims handling. This enables insurers to focus on strategic initiatives. For instance, companies like Lemonade have used chatbots to process claims in under five seconds, enhancing customer satisfaction while reducing administrative costs by up to 30%.
Potential Concern: High setup costs can deter smaller players.
Solution: Cloud-based platforms and InsurTech partnerships significantly lower the entry barrier, making advanced solutions accessible to insurers of all sizes.
3. Personalized Policies
Insurers can now tailor offerings based on customer-specific data, such as driving habits, health metrics, or operational needs. For example, telematics systems monitor driving behavior, rewarding safe drivers with lower premiums. Studies show that such usage-based pricing models can reduce claim rates by up to 20% while improving customer retention.
Potential Concern: Privacy concerns arise with extensive data collection.
Solution: Secure encryption, anonymized data processing, and adherence to regulations like GDPR help ensure customer confidence.
4. Enhanced Fraud Detection
Fraudulent claims cost the global insurance industry billions annually. Machine-driven analytics can detect anomalies and patterns indicative of fraud, helping insurers take early action. For instance, some systems flag inconsistencies in claims timelines or exaggerated damages, saving insurers significant costs while maintaining trust.
Potential Concern: Automation may reduce certain job roles
Solution: Upskilling employees to analyze outputs and handle strategic tasks ensures technology complements the workforce rather than replacing it.

Applications of AI in Insurance Risk
1. Predictive Analytics for Risk Mitigation
Predictive analytics allows insurers to anticipate future risks and take progressive measures to reduce potential losses. For example, artificial intelligence models analyze historical weather patterns, satellite imagery, and real-time climate data to assess the likelihood of natural disasters such as hurricanes, floods, or wildfires. These insights enable insurers to develop targeted risk mitigation strategies, improving both underwriting accuracy and customer protection.
Example Use Case: Climate Risk Management
Property insurers use it to evaluate flood risks by combining geospatial data with climate projections. This empowers them to recommend preventive measures to policyholders, such as elevating homes, installing flood barriers, or enhancing drainage systems. By proactively addressing risks, insurers reduce claim payouts and foster greater customer trust.
2. Real-Time Dynamic Pricing
Dynamic pricing powered by technology allows insurers to adjust premiums based on real-time data, ensuring policies remain competitive while reflecting accurate risk levels. By processing continuously updated data points, such as driving behavior, health metrics, or market trends, automated intelligence enables insurers to create more tailored and fair pricing models.
Example: Health Insurance and Wearables
Wearable fitness trackers provide health insurers with real-time data on physical activity, heart rate, and sleep patterns. AI systems analyze this data to adjust premiums dynamically, rewarding healthy behaviors with lower rates. Additionally, this approach promotes wellness initiatives, with policyholders reporting improved health outcomes due to incentives tied to wearable technology.
3. Enhanced Customer Experience
Generative ai driven chatbots, virtual assistants improve customer interactions by providing instant responses to queries, personalized policy recommendations, and real-time claims updates. These systems leverage natural language processing (NLP) to handle inquiries efficiently, ensuring consistent and reliable customer support.
Example: Virtual Assistants in Insurance
Chatbots equipped with advanced NLP can manage complex customer questions, freeing up human agents to focus on more critical tasks. This technology has shown to increase operational efficiency by as much as 60% in some insurance firms. Moreover, virtual assistants can proactively recommend coverage upgrades or additional policies based on customer profiles, enhancing cross-selling opportunities and overall satisfaction.
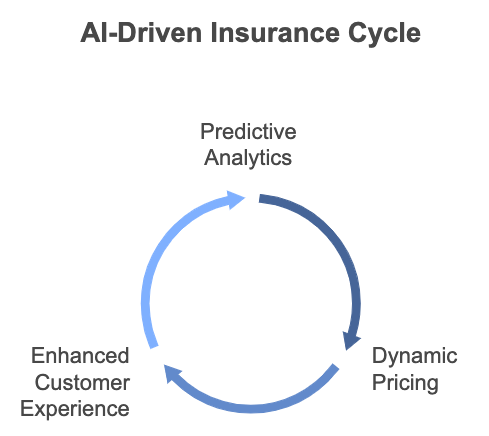
Challenges in Implementing AI-Driven Risk Initiatives
While ai risk initiatives offers transformative benefits, its adoption in the insurance industry is not without challenges. Insurers must address these hurdles to fully unlock its potential while maintaining ethical and legal compliance.
1. Data Privacy and Security
Artificial intelligence systems depend on vast amounts of sensitive personal data, such as health records, driving behavior, and financial histories. This reliance raises significant concerns about how data is collected, stored, and utilized. Additionally, insurers must navigate strict data protection regulations like the General Data Protection Regulation (GDPR) in Europe and the Health Insurance Portability and Accountability Act (HIPAA) in the United States, which impose stringent requirements on handling personal information. Failing to comply with these regulations can result in severe financial penalties and reputational damage.
Solutions for Data Security: Insurers can implement robust encryption protocols to secure sensitive data during storage and transmission. Anonymization techniques allow companies to process data without exposing personal identifiers, significantly reducing privacy risks. Furthermore, fostering transparency by clearly communicating data usage policies to customers builds trust and ensures compliance with regulations. Companies should also establish data governance frameworks to monitor and control how data is accessed and used within their organizations.
2. Algorithmic Bias
AI models are only as unbiased as the data they are trained on. If training datasets reflect historical biases or lack diversity, systems can perpetuate or even amplify these biases, leading to discriminatory outcomes. In the insurance industry, this could manifest in unfair pricing models or exclusions that disproportionately affect certain demographic groups. Such outcomes not only harm affected individuals but also erode public trust in AI technologies.
Addressing Bias in AI: Regular audits of smart algorithms are essential to identify and mitigate biases and vulnerability in decision-making processes. Insurers should prioritize training models on diverse and representative datasets to ensure equitable outcomes across all demographic groups. Additionally, incorporating ethical guidelines into AI development processes, such as fairness standards and accountability measures, can help mitigate bias. Collaboration with external organizations specializing in AI governance can further strengthen insurers’ efforts to create fair and trustworthy systems.
3. High Costs of Implementation
The initial costs of implementing and to use AI systems can be prohibitive, particularly for smaller insurers. Expenses include investments in infrastructure, advanced software, and skilled professionals to develop and maintain AI-driven solutions. Furthermore, the integration of artificial intelligence into existing workflows often requires significant time and resources, creating additional barriers for companies operating with limited budgets.
Example: Cloud Solutions:
Cloud-based platforms like Google Cloud and Amazon Web Services (AWS) offer scalable tools that significantly reduce upfront costs. These platforms enable insurers to adopt AI capabilities on a subscription basis, eliminating the need for extensive in-house infrastructure. InsurTech partnerships also provide access to affordable and ready-to-use AI tools, making the technology more accessible to smaller firms. Over time, the efficiency gains and cost savings delivered by AI's often outweigh the initial investment, making it a worthwhile long-term strategy.
4. Organizational Resistance to Change
Introducing technology-driven risk assessment to manage risk often requires a cultural shift within organizations, as employees and decision-makers may resist changes to traditional processes. Concerns about job displacement and the perceived complexity of advanced algorithmic technologies can hinder adoption. Additionally, a lack of in-house expertise may lead to delays in implementing smart computational solutions effectively.
Overcoming Resistance: Insurers can address resistance by offering training programs to upskill employees, enabling them to work alongside intelligent systems and understand their value. Clearly communicating the benefits of these technological tools to stakeholders, such as increased efficiency and improved customer experiences, can also foster acceptance. Encouraging a collaborative approach that involves employees in algorithmic implementation decisions ensures a smoother transition and reduces resistance.
By addressing these challenges proactively, insurers can leverage smart data technologies to their fullest potential while maintaining ethical practices, ensuring compliance, and fostering innovation in the insurance industry.

The Future of AI-Driven Risk Assessment
As computational technology continues to evolve, its impact on insurance risk assessment will be transformative, enabling companies to deliver smarter, faster, and more customized solutions. This includes addressing risks across supply chains, where advanced systems can analyze vulnerabilities, predict disruptions, and ensure more accurate underwriting. Below are key trends shaping the future of intelligent systems in this critical sector:
1. Tackling New and Emerging Risks
Advanced algorithms will play a pivotal role in addressing rapidly evolving threats:
Cybersecurity Risks: Sophisticated intelligent models will predict and mitigate cyberattacks, offering tailored cybersecurity policies.
Climate Change: Predictive analytics will model extreme weather patterns, helping insurers assess environmental risks with unprecedented accuracy.
Shifting Demographics: Smart computational tools will enable insurers to adapt coverage plans to suit changing demographics and lifestyles.
2. Democratization of Smart Technologies
The accessibility of algorithmic tools is levelling the playing field for insurers:
Cost-Effective Solutions: Advances in technically advanced platforms are lowering barriers to entry for smaller insurers.
Simplified Adoption: Intuitive, user-friendly intelligent systems allow smaller players to harness predictive analytics, fraud detection, and personalized customer service.
Enhanced Competition: By democratizing computational capabilities, smaller firms can offer services comparable to those of industry giants, fostering innovation and competitive pricing.
3. Integration with Emerging Technologies
Intelligent systems will converge with other cutting-edge technologies, creating a more seamless and efficient insurance ecosystem:
Blockchain: By leveraging blockchain, advanced algorithms can enhance data security and transparency in claims processing, reducing fraud and improving trust.
IoT (Internet of Things): Real-time data from IoT devices such as smart home systems, wearables, and connected vehicles will allow computational models to deliver highly accurate risk assessments and personalized policy pricing.
Cloud Computing: Machine learning's integration with cloud platforms will enable insurers to scale operations while providing instant analytics and improved customer service.
4. Hyper-Personalized Customer Experiences
Smart computational systems will redefine customer engagement in the insurance industry:
Behavioral Analysis: Intelligent algorithms can analyze consumer behavior patterns to recommend coverage that aligns with individual needs and preferences.
Dynamic Policy Pricing: Usage-based insurance (UBI) models will thrive, as computational tools leverage data from IoT devices to adjust premiums in real time.
Predictive Services: Smart chatbots and virtual assistants will provide immediate responses to queries, increasing customer satisfaction and loyalty.
5. Regulatory and Ethical Challenges
The rise of intelligent technologies in insurance will bring its own challenges, particularly in the realms of:
Regulatory Compliance: Insurers will need to navigate evolving legal frameworks to ensure algorithmic systems are fair, transparent, and unbiased.
Data Privacy: With increasing reliance on personal data, natural language processing systems must comply with stringent data protection regulations like GDPR and CCPA.
Ethical Considerations: Insurers must prioritize building responsible computational systems to avoid potential discrimination or misuse of sensitive information.
Conclusion: The future of intelligent technologies in insurance risk assessment is undeniably promising. By embracing innovation, insurers can improve efficiency, better address emerging risks, and offer more personalized services. However, the success of these advancements will hinge on responsible implementation, regulatory alignment, and continued investment in cutting-edge technology.
Key Takeaways
AI Enhances Accuracy, Efficiency, and Personalization: Artificial intelligence is revolutionizing insurance risk assessment by providing more precise data analysis, streamlining operational processes, and delivering tailored customer experiences. This transformation is reshaping the insurance landscape.
Challenges Require Proactive Solutions: Critical concerns such as data privacy, regulatory compliance, and algorithmic bias must be addressed to build trust and ensure fair outcomes. Proactive governance and responsible AI practices are essential to maintaining public confidence.
Democratization of AI Tools: The increasing availability of affordable and intuitive AI tools will empower insurers of all sizes, enabling smaller players to compete effectively. This democratization allows the industry to better tackle emerging risks such as cyberattacks and climate-related events.
Adaptation to an Evolving Risk Environment: By leveraging AI-driven insights, insurers can stay agile, anticipate shifting customer needs, and navigate the complexities of an evolving risk landscape with greater confidence.
Final Thought: Embracing responsible machine learning is not merely a technological enhancement; it is a strategic necessity for insurers striving to stay relevant in an ever-evolving landscape. By thoughtfully implementing solutions with the guidance of industry experts like LevlUp.ai and utilizing reliable and trustworthy AI systems, the industry can achieve a balance between innovation and responsibility, paving the way for a future-ready insurance ecosystem.
Next Step: Take the initiative to enhance your organization’s approach to responsible AI.
Schedule Your Readiness and Strategy Session Today!
Comments